Getting started with astir¶
Table of Contents:¶
0. Load necessary libraries¶
[2]:
# !pip install -e ../../..
import os
import sys
module_path = os.path.abspath(os.path.join('../../..'))
if module_path not in sys.path:
sys.path.append(module_path)
[4]:
from astir.data import from_csv_yaml
import pandas as pd
import numpy as np
import matplotlib.pyplot as plt
import seaborn as sns
%load_ext autoreload
%autoreload 2
%matplotlib inline
The autoreload extension is already loaded. To reload it, use:
%reload_ext autoreload
1. Load data¶
We start by reading expression data in the form of a csv file and marker gene information in the form of a yaml file:
[18]:
expression_mat_path = "../../../astir/tests/test-data/sce.csv"
# expression_mat_path = "data/sample_data.csv"
yaml_marker_path = "../../../astir/tests/test-data/jackson-2020-markers.yml"
Note
Expression data should already be cleaned and normalized. In our workflow, we perform this by a log-transformation of the data with a pseudocount of 1 (i.e. log(x+1)
), followed by winsorization at the (1%,99%) percentiles.
We can view both the expression data and marker data:
[22]:
!head -n 20 ../../../astir/tests/test-data/jackson-2020-markers.yml
cell_states:
RTK_signalling:
- Her2
- EGFR
cell_growth:
- Ki-67
- phospho mTOR
mTOR_signalling:
- phospho mTOR
- phospho S6
apoptosis:
- cleaved PARP
- Cleaved Caspase3
cell_types:
stromal:
- Vimentin
- Fibronectin
B cells:
- CD45
[7]:
pd.read_csv(expression_mat_path, index_col=0)[['EGFR','E-Cadherin', 'CD45', 'Cytokeratin 5']].head()
[7]:
EGFR | E-Cadherin | CD45 | Cytokeratin 5 | |
---|---|---|---|---|
BaselTMA_SP41_186_X5Y4_3679 | 0.346787 | 0.938354 | 0.227730 | 0.095283 |
BaselTMA_SP41_153_X7Y5_246 | 0.833752 | 1.364884 | 0.068526 | 0.124031 |
BaselTMA_SP41_20_X12Y5_197 | 0.110006 | 0.177361 | 0.301222 | 0.052750 |
BaselTMA_SP41_14_X1Y8_84 | 0.282666 | 1.122174 | 0.606941 | 0.093352 |
BaselTMA_SP41_166_X15Y4_266 | 0.209066 | 0.402554 | 0.588273 | 0.064545 |
Then we can create an astir object using the from_csv_yaml
function. For more data loading options, see the data loading tutorial.
[23]:
ast = from_csv_yaml(expression_mat_path, marker_yaml=yaml_marker_path)
print(ast)
Astir object with 6 cell types, 4 cell states, and 4931 cells.
2. Fitting cell types¶
To fit cell types, simply call
[24]:
ast.fit_type(max_epochs=10, n_init=3, n_init_epochs=2)
training restart 1/5: 100%|██████████| 5/5 [ 3.76s/epochs, current loss: -1693.0]
training restart 2/5: 100%|██████████| 5/5 [ 4.03s/epochs, current loss: 1273.4]
training restart 3/5: 100%|██████████| 5/5 [ 3.54s/epochs, current loss: -640.5]
training restart 4/5: 100%|██████████| 5/5 [ 3.41s/epochs, current loss: 198.5]
training restart 5/5: 100%|██████████| 5/5 [ 3.44s/epochs, current loss: -415.8]
training restart (final): 94%|█████████▍| 47/50 [ 3.51s/epochs, current loss: -17252.6]
Note
Controlling inference There are many different options for controlling inference in the fit_type
function, including max_epochs
(maximum number of epochs to train), learning_rate
(ADAM optimizer learning rate), batch_size
(minibatch size), delta_loss
(stops iteration once the change in loss falls below this value), n_inits
(number of restarts using random initializations). For full details, see the function documentation.
We should always plot the losses to assess convergence:
[25]:
plt.figure(figsize=(5,4))
plt.plot(np.arange(len(ast.get_type_losses())), ast.get_type_losses())
plt.ylabel("Loss")
plt.xlabel("Epoch")
[25]:
Text(0.5, 0, 'Epoch')
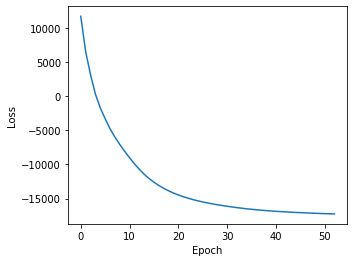
We can then get cell type assignment probabilities by calling
[26]:
assignments = ast.get_celltype_probabilities()
assignments
[26]:
stromal | B cells | T cells | macrophage | epithelial(basal) | epithelial(luminal) | Other | |
---|---|---|---|---|---|---|---|
BaselTMA_SP43_115_X4Y8_1 | 5.981264e-05 | 4.351427e-01 | 4.413378e-06 | 4.778704e-05 | 0.005910 | 0.000002 | 5.588340e-01 |
BaselTMA_SP43_115_X4Y8_2 | 7.080264e-05 | 3.849646e-01 | 5.889273e-06 | 4.314298e-05 | 0.044710 | 0.000007 | 5.701980e-01 |
BaselTMA_SP43_115_X4Y8_3 | 2.086748e-04 | 1.871444e-01 | 9.592506e-04 | 4.061270e-04 | 0.290781 | 0.039960 | 4.805403e-01 |
BaselTMA_SP43_115_X4Y8_4 | 2.980559e-04 | 4.438626e-01 | 6.048962e-06 | 1.707915e-04 | 0.011950 | 0.000006 | 5.437063e-01 |
BaselTMA_SP43_115_X4Y8_5 | 1.121071e-04 | 4.681895e-01 | 2.136392e-05 | 3.064268e-05 | 0.017083 | 0.000002 | 5.145617e-01 |
... | ... | ... | ... | ... | ... | ... | ... |
BaselTMA_SP43_115_X4Y8_4927 | 4.338320e-07 | 1.912927e-03 | 2.400906e-07 | 1.376804e-06 | 0.993716 | 0.000003 | 4.365914e-03 |
BaselTMA_SP43_115_X4Y8_4928 | 2.193680e-04 | 3.908094e-01 | 3.504072e-04 | 2.164537e-04 | 0.009062 | 0.000018 | 5.993251e-01 |
BaselTMA_SP43_115_X4Y8_4929 | 1.189970e-08 | 7.369497e-07 | 5.310283e-09 | 8.920690e-09 | 0.999534 | 0.000464 | 8.322494e-07 |
BaselTMA_SP43_115_X4Y8_4930 | 5.812946e-08 | 6.018526e-09 | 2.364501e-08 | 5.991192e-09 | 0.000590 | 0.999410 | 6.340690e-08 |
BaselTMA_SP43_115_X4Y8_4931 | 1.341373e-05 | 1.754594e-06 | 2.443332e-06 | 8.300306e-07 | 0.060859 | 0.939114 | 8.438225e-06 |
4931 rows × 7 columns
We can also visualize the assignment probabilities using a heatmap:
[27]:
sns.heatmap(assignments)
[27]:
<matplotlib.axes._subplots.AxesSubplot at 0x7f8d611c72d0>
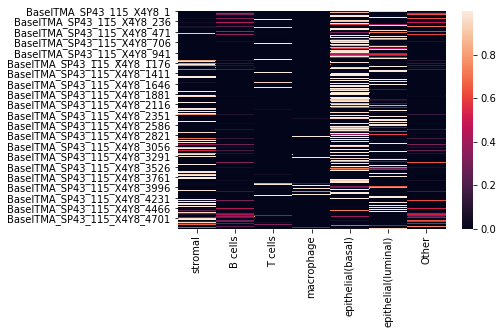
where each row corresponds to a cell, and each column to a cell type, with the entry being the probability of that cell belonging to a particular cell type.
To fetch an array corresponding to the most likely cell type assignments, call
[28]:
ast.get_celltypes()
[28]:
cell_type | |
---|---|
BaselTMA_SP43_115_X4Y8_1 | Unknown |
BaselTMA_SP43_115_X4Y8_2 | Unknown |
BaselTMA_SP43_115_X4Y8_3 | Unknown |
BaselTMA_SP43_115_X4Y8_4 | Unknown |
BaselTMA_SP43_115_X4Y8_5 | Unknown |
... | ... |
BaselTMA_SP43_115_X4Y8_4927 | epithelial(basal) |
BaselTMA_SP43_115_X4Y8_4928 | Unknown |
BaselTMA_SP43_115_X4Y8_4929 | epithelial(basal) |
BaselTMA_SP43_115_X4Y8_4930 | epithelial(luminal) |
BaselTMA_SP43_115_X4Y8_4931 | epithelial(luminal) |
4931 rows × 1 columns
Cell type diagnostics¶
It is important to run diagnostics to ensure that cell types express their markers at higher levels than other cell types. To do this, run the diagnostics_celltype()
function, which will alert to any issues if a cell type doesn’t express its marker signficantly higher than an alternative cell type (for which that protein isn’t a marker):
[29]:
ast.diagnostics_celltype().head(n=10)
[29]:
feature | should be expressed higher in | than | mean cell type 1 | mean cell type 2 | p-value | note | |
---|---|---|---|---|---|---|---|
0 | Fibronectin | stromal | B cells | 2.045195 | 1.565420 | inf | Only 1 cell in a type: comparison not possible |
1 | Vimentin | stromal | B cells | 2.994335 | 1.095477 | inf | Only 1 cell in a type: comparison not possible |
2 | CD20 | B cells | stromal | 0.403677 | 0.081944 | inf | Only 1 cell in a type: comparison not possible |
3 | CD20 | B cells | T cells | 0.403677 | 0.123195 | inf | Only 1 cell in a type: comparison not possible |
4 | CD20 | B cells | macrophage | 0.403677 | 0.212008 | inf | Only 1 cell in a type: comparison not possible |
5 | CD20 | B cells | epithelial(basal) | 0.403677 | 0.118228 | inf | Only 1 cell in a type: comparison not possible |
6 | CD20 | B cells | epithelial(luminal) | 0.403677 | 0.131991 | inf | Only 1 cell in a type: comparison not possible |
7 | CD20 | B cells | Other | 0.403677 | 0.017356 | inf | Only 1 cell in a type: comparison not possible |
8 | CD45 | B cells | stromal | 0.077596 | 0.227257 | inf | Only 1 cell in a type: comparison not possible |
9 | CD45 | B cells | epithelial(basal) | 0.077596 | 0.182078 | inf | Only 1 cell in a type: comparison not possible |
Note
In this tutorial, we end up with many “Only 1 cell in a type: comparison not possible” notes - this is simply because the small dataset size results in only a single cell assigned to many types, making statistical testing infeasible.
Calling ast.diagnostics_celltype()
returns a pd.DataFrame
, where each column corresponds to a particular protein and two cell types, with a warning if the protein is not expressed at higher levels in the cell type for which it is a marker than the cell type for which it is not.
The diagnostics:
Iterates through every cell type and every marker for that cell type
Given a cell type c and marker g, find the set of cell types D that don’t have g as a marker
For each cell type d in D, perform a t-test between the expression of marker g in c vs d
If g is not expressed significantly higher (at significance alpha), output a diagnostic explaining this for further investigation.
If multiple issues are found, the markers and cell types may need refined.
3. Fitting cell state¶
Similarly as before, to fit cell state, call
[30]:
ast.fit_state(batch_size = 1024, learning_rate=1e-3, max_epochs=10)
/Users/jinelles.h/Documents/Camlab/astir-top-level/astir/astir/models/cellstate.py:176: UserWarning: Delta loss batch size is greater than the number of epochs
warnings.warn("Delta loss batch size is greater than the number of epochs")
training restart 1/5: 100%|██████████| 5/5 [ 3.41s/epochs, current loss: 59.8]
training restart 2/5: 100%|██████████| 5/5 [ 3.40s/epochs, current loss: 98.0]
training restart 3/5: 100%|██████████| 5/5 [ 3.51s/epochs, current loss: 78.2]
training restart 4/5: 100%|██████████| 5/5 [ 3.48s/epochs, current loss: 88.0]
training restart 5/5: 100%|██████████| 5/5 [ 3.37s/epochs, current loss: 60.0]
training restart (final): 100%|██████████| 50/50 [ 3.60s/epochs, current loss: 14.5]
/Users/jinelles.h/Documents/Camlab/astir-top-level/astir/astir/astir.py:268: UserWarning: Maximum epochs reached. More iteration may be needed to complete the training.
warnings.warn(msg)
and similary plot the losses via
[31]:
plt.figure(figsize=(5,4))
plt.plot(np.arange(len(ast.get_state_losses())), ast.get_state_losses())
plt.ylabel("Loss")
plt.xlabel("Epoch")
[31]:
Text(0.5, 0, 'Epoch')
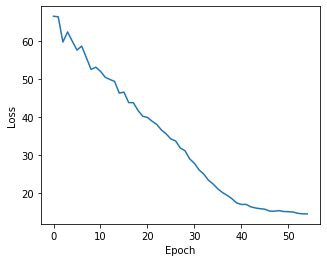
and cell state assignments can be inferred via
[32]:
states = ast.get_cellstates()
states
[32]:
RTK_signalling | cell_growth | mTOR_signalling | apoptosis | |
---|---|---|---|---|
BaselTMA_SP43_115_X4Y8_1 | 0.219178 | 0.178849 | 0.133436 | 0.144105 |
BaselTMA_SP43_115_X4Y8_2 | 0.296468 | 0.119678 | 0.200147 | 0.157937 |
BaselTMA_SP43_115_X4Y8_3 | 0.181820 | 0.185739 | 0.117271 | 0.117391 |
BaselTMA_SP43_115_X4Y8_4 | 0.271369 | 0.091272 | 0.145709 | 0.170535 |
BaselTMA_SP43_115_X4Y8_5 | 0.238632 | 0.219416 | 0.147383 | 0.165110 |
... | ... | ... | ... | ... |
BaselTMA_SP43_115_X4Y8_4927 | 0.377885 | 0.229766 | 0.257093 | 0.232393 |
BaselTMA_SP43_115_X4Y8_4928 | 0.144620 | 0.335371 | 0.105722 | 0.134628 |
BaselTMA_SP43_115_X4Y8_4929 | 0.494012 | 0.387052 | 0.286395 | 0.357899 |
BaselTMA_SP43_115_X4Y8_4930 | 0.624909 | 0.492357 | 0.315533 | 0.519452 |
BaselTMA_SP43_115_X4Y8_4931 | 0.631358 | 0.453455 | 0.292822 | 0.536574 |
4931 rows × 4 columns
[33]:
plt.scatter(
states['RTK_signalling'],
ast.get_state_dataset().get_exprs_df()['Her2']
)
[33]:
<matplotlib.collections.PathCollection at 0x7f8d60937b90>
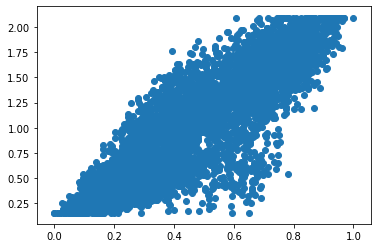
Cell state diagnostics¶
It is important to run diagnostics on cell states model for the same reasons stated for the cell type model. Astir.diagnostics_cellstate()
spots any non marker protein and pathway pairs whose expressions are higher than those of the marker proteins of the pathway.
[34]:
ast.diagnostics_cellstate().head(n=10)
[34]:
pathway | protein A | correlation of protein A | protein B | correlation of protein B | note | |
---|---|---|---|---|---|---|
0 | RTK_signalling | EGFR | 0.823298 | Cleaved Caspase3 | 0.831128 | EGFR is marker for RTK_signalling but Cleaved ... |
1 | RTK_signalling | EGFR | 0.823298 | cleaved PARP | 0.831128 | EGFR is marker for RTK_signalling but cleaved ... |
2 | cell_growth | Ki-67 | 0.058879 | Cleaved Caspase3 | 0.862459 | Ki-67 is marker for cell_growth but Cleaved Ca... |
3 | cell_growth | Ki-67 | 0.058879 | EGFR | 0.897650 | Ki-67 is marker for cell_growth but EGFR isn't |
4 | cell_growth | Ki-67 | 0.058879 | Her2 | 0.750985 | Ki-67 is marker for cell_growth but Her2 isn't |
5 | cell_growth | Ki-67 | 0.058879 | cleaved PARP | 0.862459 | Ki-67 is marker for cell_growth but cleaved PA... |
6 | cell_growth | Ki-67 | 0.058879 | phospho S6 | 0.272989 | Ki-67 is marker for cell_growth but phospho S6... |
7 | mTOR_signalling | phospho S6 | 0.699387 | EGFR | 0.720013 | phospho S6 is marker for mTOR_signalling but E... |
8 | mTOR_signalling | phospho S6 | 0.699387 | Her2 | 0.757867 | phospho S6 is marker for mTOR_signalling but H... |
Calling ast.diagnostics_cellstate()
returns a pd.DataFrame
, where each column corresponds to a particular protein and two cell types, with a warning if the protein is not expressed at higher levels in the cell state for which it is a marker than the cell state for which it is not.
The diagnostics:
Get correlations between all cell states and proteins
For each cell state c, get the smallest correlation with marker g
For each cell state c and its non marker g, find any correlation that is bigger than those smallest correlation for c.
Any c and g pairs found in step 3 will be included in the output of
Astir.diagnostics_cellstate()
, including an explanation.
If multiple issues are found, the markers and cell states may need refined.
4. Saving results¶
Both cell type and cell state information can easily be saved to disk via
[35]:
ast.type_to_csv("data/cell-types.csv")
ast.state_to_csv("data/cell-states.csv")
[37]:
!head -n 3 data/cell-types.csv
,stromal,B cells,T cells,macrophage,epithelial(basal),epithelial(luminal),Other
BaselTMA_SP43_115_X4Y8_1,5.9812641356773485e-05,0.43514272442399615,4.4133780759206555e-06,4.778704083811438e-05,0.00590960852651017,1.6351770806453748e-06,0.5588340188121422
BaselTMA_SP43_115_X4Y8_2,7.080264289768121e-05,0.38496458175145926,5.889272783675721e-06,4.3142984449199766e-05,0.04471036731616506,7.2442437475747445e-06,0.5701979717884975
[38]:
!head -n 3 data/cell-states.csv
,RTK_signalling,cell_growth,mTOR_signalling,apoptosis
BaselTMA_SP43_115_X4Y8_1,0.2191776340692214,0.17884886731101723,0.13343558969907734,0.1441052515306596
BaselTMA_SP43_115_X4Y8_2,0.29646752007327026,0.11967817733248733,0.20014708539822942,0.1579368118796618
where the first (unnamed) column always corresponds to the cell name/ID.
5. Accessing internal functions and data¶
Data stored in astir
objects is in the form of an SCDataSet
. These can be retrieved via
[39]:
celltype_data = ast.get_type_dataset()
celltype_data
[39]:
<astir.data.scdataset.SCDataset at 0x7f8d60bd6ed0>
and similarly for cell state via ast.get_state_dataset()
.
These have several helper functions to retrieve relevant information to the dataset:
[40]:
celltype_data.get_cell_names()[0:4] # cell names
[40]:
['BaselTMA_SP43_115_X4Y8_1',
'BaselTMA_SP43_115_X4Y8_2',
'BaselTMA_SP43_115_X4Y8_3',
'BaselTMA_SP43_115_X4Y8_4']
[41]:
celltype_data.get_classes() # cell type names
[41]:
['stromal',
'B cells',
'T cells',
'macrophage',
'epithelial(basal)',
'epithelial(luminal)']
[42]:
print(celltype_data.get_n_classes()) # number of cell types
print(celltype_data.get_n_features()) # number of features / proteins
6
14
[43]:
celltype_data.get_exprs() # Return a torch tensor corresponding to the expression data used
[43]:
tensor([[4.2121e-02, 5.2044e-02, 1.7348e-03, ..., 6.1314e-01, 4.4827e-02,
3.8841e-01],
[0.0000e+00, 3.4770e-02, 0.0000e+00, ..., 9.4025e-01, 1.9424e-02,
5.6716e-01],
[1.8200e-02, 7.8596e-02, 0.0000e+00, ..., 6.2852e-01, 4.0905e-02,
8.4946e-01],
...,
[1.4403e-01, 7.0877e-02, 2.0325e-01, ..., 1.7303e+00, 2.1434e-01,
9.4889e-01],
[3.6400e-02, 9.9307e-02, 1.1815e-01, ..., 1.6467e+00, 1.3463e-01,
1.9238e+00],
[5.4069e-02, 1.1861e-01, 5.3894e-02, ..., 1.4265e+00, 4.9443e-01,
1.6126e+00]], dtype=torch.float64)
[44]:
celltype_data.get_exprs_df() # Return a pandas DataFrame corresponding to the expression data used
[44]:
CD20 | CD3 | CD45 | CD68 | Cytokeratin 14 | Cytokeratin 19 | Cytokeratin 5 | Cytokeratin 7 | Cytokeratin 8/18 | E-Cadherin | Fibronectin | Her2 | Vimentin | pan Cytokeratin | |
---|---|---|---|---|---|---|---|---|---|---|---|---|---|---|
BaselTMA_SP43_115_X4Y8_1 | 0.042121 | 0.052044 | 0.001735 | 0.042628 | 0.046076 | 0.076746 | 0.113790 | 0.073571 | 0.110530 | 0.800019 | 0.324533 | 0.613138 | 0.044827 | 0.388409 |
BaselTMA_SP43_115_X4Y8_2 | 0.000000 | 0.034770 | 0.000000 | 0.084581 | 0.059064 | 0.056487 | 0.098873 | 0.019242 | 0.152825 | 0.972369 | 0.384034 | 0.940250 | 0.019424 | 0.567159 |
BaselTMA_SP43_115_X4Y8_3 | 0.018200 | 0.078596 | 0.000000 | 0.042628 | 0.026254 | 0.126815 | 0.165966 | 0.310404 | 0.253835 | 1.108996 | 0.256832 | 0.628524 | 0.040905 | 0.849462 |
BaselTMA_SP43_115_X4Y8_4 | 0.000000 | 0.018701 | 0.015682 | 0.108294 | 0.071382 | 0.052757 | 0.022484 | 0.093607 | 0.062275 | 1.204820 | 0.661950 | 0.530076 | 0.093848 | 0.418222 |
BaselTMA_SP43_115_X4Y8_5 | 0.099789 | 0.073795 | 0.093230 | 0.122463 | 0.000000 | 0.238924 | 0.193627 | 0.000000 | 0.125397 | 1.225161 | 0.623664 | 0.493992 | 0.000000 | 0.296923 |
... | ... | ... | ... | ... | ... | ... | ... | ... | ... | ... | ... | ... | ... | ... |
BaselTMA_SP43_115_X4Y8_4927 | 0.000000 | 0.036476 | 0.142845 | 0.384770 | 0.046530 | 0.000362 | 0.139654 | 0.000000 | 0.000000 | 1.730274 | 0.233316 | 1.276169 | 0.171234 | 0.396967 |
BaselTMA_SP43_115_X4Y8_4928 | 0.000000 | 0.100622 | 0.089643 | 0.091315 | 0.000000 | 0.098437 | 0.000000 | 0.000000 | 0.155443 | 0.908461 | 0.411734 | 0.365833 | 0.110548 | 0.789458 |
BaselTMA_SP43_115_X4Y8_4929 | 0.144029 | 0.070877 | 0.203246 | 0.503439 | 0.051248 | 0.200929 | 0.138156 | 0.045556 | 0.057138 | 2.136403 | 0.841147 | 1.730263 | 0.214340 | 0.948885 |
BaselTMA_SP43_115_X4Y8_4930 | 0.036400 | 0.099307 | 0.118155 | 0.447235 | 0.285294 | 0.270529 | 0.107387 | 0.423265 | 0.234452 | 1.884903 | 0.767309 | 1.646707 | 0.134634 | 1.923792 |
BaselTMA_SP43_115_X4Y8_4931 | 0.054069 | 0.118611 | 0.053894 | 0.484753 | 0.340787 | 0.133814 | 0.150674 | 0.365944 | 0.163528 | 1.843372 | 1.161388 | 1.426493 | 0.494433 | 1.612622 |
4931 rows × 14 columns
[45]:
ast.normalize()
[46]:
ast.get_type_dataset().get_exprs_df()
[46]:
CD20 | CD3 | CD45 | CD68 | Cytokeratin 14 | Cytokeratin 19 | Cytokeratin 5 | Cytokeratin 7 | Cytokeratin 8/18 | E-Cadherin | Fibronectin | Her2 | Vimentin | pan Cytokeratin | |
---|---|---|---|---|---|---|---|---|---|---|---|---|---|---|
BaselTMA_SP43_115_X4Y8_1 | 0.008424 | 0.010409 | 0.000347 | 0.008586 | 0.009215 | 0.015349 | 0.022756 | 0.014714 | 0.022104 | 0.159329 | 0.064861 | 0.122322 | 0.008965 | 0.077604 |
BaselTMA_SP43_115_X4Y8_2 | 0.000000 | 0.006954 | 0.000000 | 0.016915 | 0.011813 | 0.011297 | 0.019773 | 0.003848 | 0.030560 | 0.193268 | 0.076731 | 0.186959 | 0.003885 | 0.113190 |
BaselTMA_SP43_115_X4Y8_3 | 0.003640 | 0.015719 | 0.000000 | 0.008586 | 0.005251 | 0.025360 | 0.033187 | 0.062041 | 0.050745 | 0.220020 | 0.051344 | 0.125376 | 0.008181 | 0.169086 |
BaselTMA_SP43_115_X4Y8_4 | 0.000000 | 0.003740 | 0.003136 | 0.021657 | 0.014276 | 0.010551 | 0.004497 | 0.018720 | 0.012455 | 0.238691 | 0.132006 | 0.105818 | 0.018769 | 0.083547 |
BaselTMA_SP43_115_X4Y8_5 | 0.019956 | 0.014758 | 0.018645 | 0.024490 | 0.000000 | 0.047767 | 0.038716 | 0.000000 | 0.025077 | 0.242644 | 0.124412 | 0.098638 | 0.000000 | 0.059350 |
... | ... | ... | ... | ... | ... | ... | ... | ... | ... | ... | ... | ... | ... | ... |
BaselTMA_SP43_115_X4Y8_4927 | 0.000000 | 0.007295 | 0.028565 | 0.076878 | 0.009306 | 0.000123 | 0.027927 | 0.000000 | 0.000000 | 0.339496 | 0.046690 | 0.252541 | 0.034240 | 0.079310 |
BaselTMA_SP43_115_X4Y8_4928 | 0.000000 | 0.020123 | 0.017928 | 0.018262 | 0.000000 | 0.019686 | 0.000000 | 0.000000 | 0.031084 | 0.180707 | 0.082254 | 0.073101 | 0.022108 | 0.157243 |
BaselTMA_SP43_115_X4Y8_4929 | 0.028802 | 0.014175 | 0.040638 | 0.100518 | 0.010249 | 0.040175 | 0.027628 | 0.009111 | 0.011427 | 0.415244 | 0.167446 | 0.339493 | 0.042855 | 0.188656 |
BaselTMA_SP43_115_X4Y8_4930 | 0.007280 | 0.019860 | 0.023629 | 0.089328 | 0.057028 | 0.054079 | 0.021476 | 0.084552 | 0.046873 | 0.368578 | 0.152866 | 0.323661 | 0.026924 | 0.375847 |
BaselTMA_SP43_115_X4Y8_4931 | 0.010813 | 0.023720 | 0.010779 | 0.096799 | 0.068105 | 0.026760 | 0.030130 | 0.073124 | 0.032700 | 0.360796 | 0.230238 | 0.281564 | 0.098726 | 0.317179 |
4931 rows × 14 columns
6. Saving models¶
After fixing the models, we can save the cell type/state assignment, the losses, the parameters (e.g. mu
, rho
, log_sigma
, etc) and the run informations (e.g. batch_size
, learning_rate
, delta_loss
, etc) to an hdf5 file.
[48]:
ast.save_models("data/astir_summary.hdf5")
The hierarchy of the hdf5 file would be:
Only the model that is trained will be saved (CellTypeModel
or CellStateModel
or both). If the functioned is called before any model is trained, exception will be raised. Data saved in the file is either int
or np.array
.
7. Plot clustermap of expression data¶
After fixing the cell type model, we can also plot a heatmap of protein expression of cells clustered by type. The heatmap will be saved at the location plot_name
, which is default to "./celltype_protein_cluster.png"
[50]:
ast.type_clustermap(plot_name="./img/celltype_protein_cluster.png", threshold = 0.7, figsize=(7, 5))
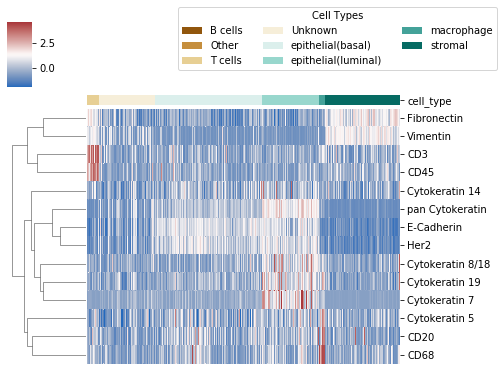
Note: threshold
is the probability threshold above which a cell is assigned to a cell type, default to 0.7.
8. Hierarchical model specification¶
In the marker yaml file, the user can also add a section called hierarchy
, which specifies the hierarchical structure of cell types. Here’s an example:
hierarchy:
immune:
- B cells
- T cells
- macrophage
epithelial:
- epithelial(basal)
- epithelial(luminal)
Some notes: 1. The section would be accessed by key hierarchy
. 2. In the section, the higher-levelled cell type names should be the keys. 3. The values in the section should also exist as the cell type names in the cell_types
section. (e.g. if we have "B cells"
in marker["hierarchy"]["immune"]
, we should also be able to get marker["cell_types"]["B cells"]
)
This section could be used to summarize the cell types assignment at a higher hierarchical level. (e.g. a cell is predicted as “immune” instead of “B cells” or “T cells”)
[53]:
hierarchy_probs = ast.assign_celltype_hierarchy()
hierarchy_probs.head()
[53]:
immune | epithelial | |
---|---|---|
BaselTMA_SP43_115_X4Y8_1 | 0.435195 | 0.005911 |
BaselTMA_SP43_115_X4Y8_2 | 0.385014 | 0.044718 |
BaselTMA_SP43_115_X4Y8_3 | 0.188510 | 0.330741 |
BaselTMA_SP43_115_X4Y8_4 | 0.444039 | 0.011956 |
BaselTMA_SP43_115_X4Y8_5 | 0.468241 | 0.017085 |
To make it more clear, here’s a heatmap for the cell assignment in a higher hierarchy:
The way it is calculated is simply summing up the probabilities of the cell type assignments under the same hierarchy.
[ ]:
[ ]:
[ ]: